
Note: These numbers are no longer up to date, but the current potential profits have taken a nosedive, right alongside the cryptocurrency prices.
#Shark gpu mining reveiw update
The latest update uses pricing data from the month of January 2022, combined with current Ethereum prices. We periodically update this article, at least the main table showing potential profits and pricing. How good? That's what we're here to discuss, and we've got hard numbers on hashing performance, prices, power, and more. Not surprisingly, the best graphics cards and those chips at the top of our GPU benchmarks hierarchy end up being very good options for mining as well. Everyone who didn't start mining last time is kicking themselves for their lack of foresight.
#Shark gpu mining reveiw how to
How to apply this pooling correctly, have a look at sentence-transformers/bert-base-nli-max-tokens and /sentence-transformers/bert-base-nli-cls-token.What are the best mining GPUs, and is it worth getting into the whole cryptocurrency craze? Bitcoin and Ethereum mining have been making headlines again, as prices and mining profitability were way up compared to the last couple of years. We also have models with max-pooling and where we use the CLS token. In the above example we add mean pooling on top of the AutoModel (which will load a BERT model). In this case, mean pooling sentence_embeddings = mean_pooling ( model_output, encoded_input ) no_grad (): model_output = model ( ** encoded_input ) #Perform pooling. from_pretrained ( "sentence-transformers/all-MiniLM-L6-v2" ) #Tokenize sentences encoded_input = tokenizer ( sentences, padding = True, truncation = True, max_length = 128, return_tensors = 'pt' ) #Compute token embeddings with torch. from_pretrained ( "sentence-transformers/all-MiniLM-L6-v2" ) model = AutoModel. sum ( 1 ), min = 1e-9 ) return sum_embeddings / sum_mask #Sentences we want sentence embeddings for sentences = #Load AutoModel from huggingface model repository tokenizer = AutoTokenizer. sum ( token_embeddings * input_mask_expanded, 1 ) sum_mask = torch. If convert_to_numpy, a numpy matrix is returned.įrom transformers import AutoTokenizer, AutoModel import torch #Mean Pooling - Take attention mask into account for correct averaging def mean_pooling ( model_output, attention_mask ): token_embeddings = model_output #First element of model_output contains all token embeddings input_mask_expanded = attention_mask. If convert_to_tensor, a stacked tensor is returned. In that case, the faster dot-product (util.dot_score) instead of cosine similarity can be used.īy default, a list of tensors is returned. Normalize_embeddings – If set to true, returned vectors will have length 1. Overwrites any setting from convert_to_numpyĭevice – Which vice to use for the computation Else, it is a list of pytorch tensors.Ĭonvert_to_tensor – If true, you get one large tensor as return.

Set to None, to get all output valuesĬonvert_to_numpy – If true, the output is a list of numpy vectors. Can be set to token_embeddings to get wordpiece token embeddings. Output_value – Default sentence_embedding, to get sentence embeddings. Show_progress_bar – Output a progress bar when encode sentences encode ( sentences : Union ], batch_size : int = 32, show_progress_bar : Optional = None, output_value : str = 'sentence_embedding', convert_to_numpy : bool = True, convert_to_tensor : bool = False, device : Optional = None, normalize_embeddings : bool = False ) → Union, numpy.ndarray, torch.Tensor ] ¶īatch_size – the batch size used for the computation

Initializes internal Module state, shared by both nn.Module and ScriptModule.
#Shark gpu mining reveiw download
Use_auth_token – HuggingFace authentication token to download private models. Modules – This parameter can be used to create custom SentenceTransformer models from scratch.ĭevice – Device (like ‘cuda’ / ‘cpu’) that should be used for computation. If that fails, tries to construct a model from Huggingface models repository with that name. If it is not a path, it first tries to download a pre-trained SentenceTransformer model. Model_name_or_path – If it is a filepath on disc, it loads the model from that path. Loads or create a SentenceTransformer model, that can be used to map sentences / text to embeddings. SentenceTransformer ( model_name_or_path : Optional = None, modules : Optional ] = None, device : Optional = None, cache_folder : Optional = None, use_auth_token : Optional ] = None ) ¶
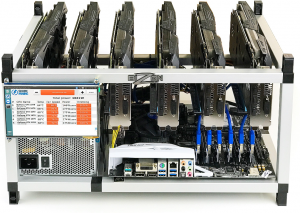
Some relevant parameters are batch_size (depending on your GPU a different batch size is optimal) as well as convert_to_numpy (returns a numpy matrix) and convert_to_tensor (returns a pytorch tensor). In the following, you can find parameters this method accepts. The relevant method to encode a set of sentences / texts is model.encode(). With device any pytorch device (like CPU, cuda, cuda:0 etc.) Model = SentenceTransformer ( 'model_name_or_path', device = 'cuda' )
